February 2025 – The RadioVal Consortium held a highly productive meeting to review progress, discuss challenges, and plan the next steps in the development of AI tools for clinical radiology.
Data Recruitment and Platform Updates
A major update was made to the data recruitment table, introducing new fields to track patient data more effectively. Clinical sites are now asked to update this table to ensure accurate data collection. The Xnat platform, designed for data storage, passed a successful upload test, and access credentials for the test project will be distributed soon.
Model Development
The consortium also shared updates on the development of several AI models:
- Automated Segmentation Models (UB): These models, initially trained on the MAMA-MIA dataset, will be retrained with new data as it becomes available.
- Image Quality Control (FORTH): A new dataset was created by artificially degrading high-quality images, overcoming challenges with limited annotated data.
- Explainability Tools (UM): Enhancements were made to model plots based on clinician feedback, improving their interpretability. These updates will be integrated into QUIBIM software.
- Human-in-the-Loop Feedback (MAG): A set of tailored questions for clinical evaluations has been developed, with a prototype of the Human Enveloped Mechanism to be ready by February’s end.
QUIBIM also presented new tools, RadioNac (a decision support system) and RadioTrack (a traceability tool), which will be essential for monitoring model performance and tracking progress.
Cost-Effectiveness and Regulatory Considerations
WP2 reported on the ongoing cost-effectiveness analysis of the RadioNac tool, with a preliminary framework now in place. The consortium also discussed the regulatory implications for prospective data and will hold a meeting in March to ensure compliance with MDR.
Next Steps
The following actions were agreed upon:
- Clinical sites will update data tables and continue integrating the Xnat platform.
- AI models will be refined based on feedback, and explainability tools will be further improved.
- Regulatory and cost-effectiveness discussions will take place in March.
A huge thank you to all consortium partners for their continued hard work and valuable contributions. Your efforts are vital to the success of this groundbreaking project, and we look forward to the next phase of collaboration.
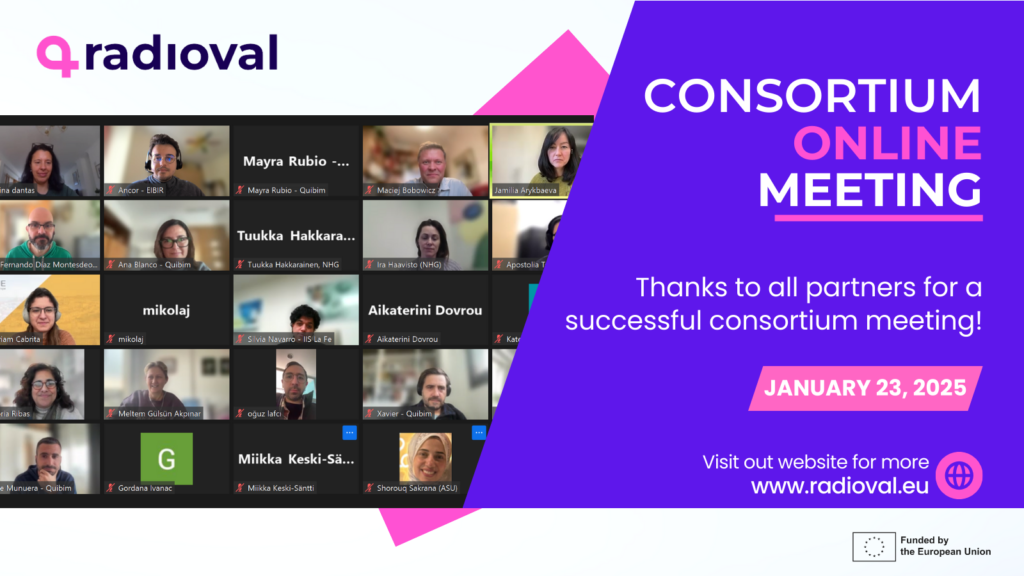